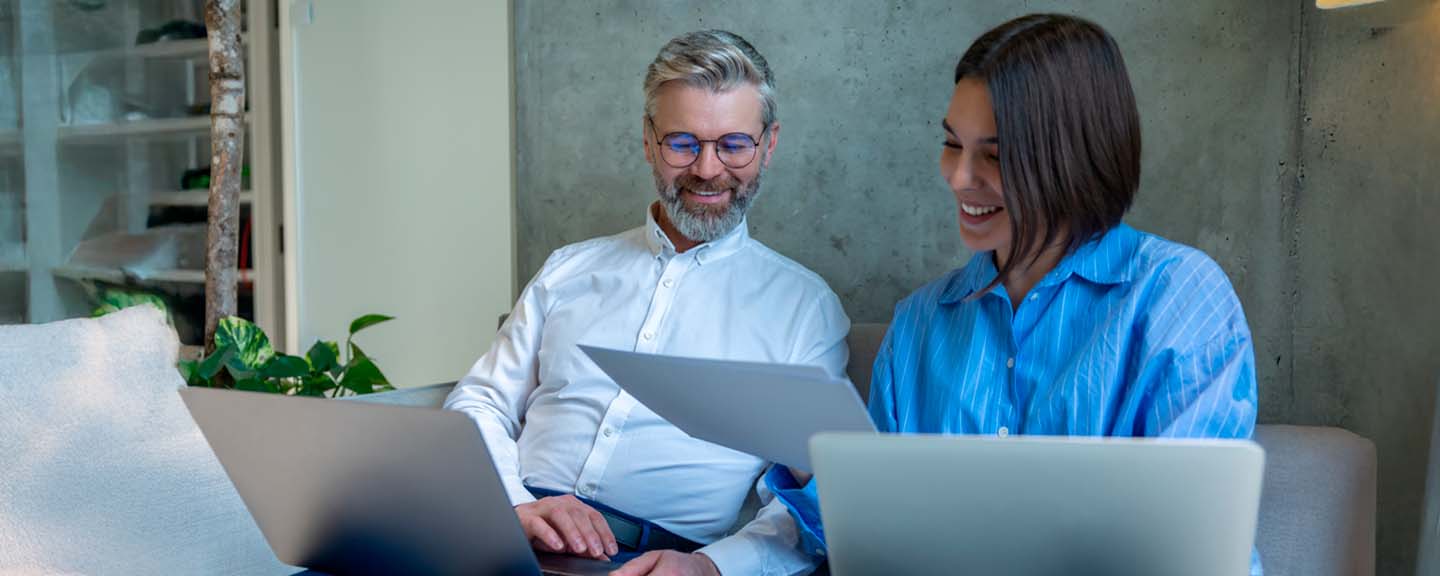
AI is having a massive impact on the way people work, and while using it obviously makes them considerably more efficient for many reasons, there is a less acknowledged issue - knowledge transfer.
The problem is one of translation. Retiring experts possess a great deal of tacit knowledge – unspoken intuition and experience-driven insights that have been refined over decades.
By no means can a large language model (LLM) replicate this knowledge because LLMs parse the world in binaries. While exceptionally powerful, these tools lack the grit of context and the “why” behind the “what”.
This article presents certain methodologies and frameworks that can enable organizations to preserve their institutional memory and leverage expert networks while also tapping into AI’s predictive capabilities.
Junior practitioners vs. C-suite veterans
Fundamentally, all institutional knowledge rests on the spectrum between junior talent and C-suite specialists who have many years of experience under their belt. It is important to highlight that each group offers unique advantages – and limitations – in capturing, structuring, and operationalizing expertise to train AI.
Junior practitioners
Definition: Early-career professionals who are immersed in daily operations, from technical workflows to emerging tools.
Strengths:
- Ground-level precision: Their hands-on experience provides AI trainers with granular data – the "how" of tasks, from coding protocols to supply-chain adjustments.
- Technical agility: They adapt swiftly to new software and AI tools, making them ideal for translating niche processes into machine-readable formats.
- Cost efficiency: Their labor is scalable for repetitive or highly specialized knowledge-capture tasks.
Weaknesses:
- Tunnel vision: By focusing on immediate tasks, they often lack context for long-term strategic involvement.
- Credibility gaps: Their input may be dismissed during high-stakes decision-making, limiting their ability to validate AI outputs for executive use.
C-Suite veterans
Definition: Retired or senior leaders with decades of strategic decision-making experience.
Strengths:
- Macro-level insight: They provide the "why" behind the historical decisions that could help AI models anticipate market shifts and stakeholder behaviour
- Authority: Their endorsement legitimizes AI systems in boardrooms to facilitate adoption.
- Network leverage: Their industry connections can source scarce data or validate AI predictions against real-world outcomes.
Weaknesses:
- Technical obsolescence: Many lack fluency with modern tools, which creates blind spots in translating their wisdom into AI-compatible formats.
- High cost: Their consultative rates put pressure on budgets, making sustained collaboration impractical for some organizations.
Juniors and veterans are complementary forces when bridging the knowledge gap, whereby the former excel at digitizing explicit knowledge – processes, technical steps, and data patterns – while the latter can provide tacit knowledge – unwritten rules, crisis navigation, and strategic trade-offs.
Without juniors, AI models lack the precision of current systems. Without veterans, they lack the foresight to avoid past mistakes.
Therefore, for businesses willing to extract knowledge before it disappears, the solution lies in a structured collaboration that pairs juniors to codify workflows with veterans to contextualize them.
Example: A retired manufacturing executive can explain why specific safety protocols evolved (strategic foresight), while a junior engineer can map how to automate these (technical execution). Together, they can create AI training datasets that are both operationally precise and strategically robust.
Expert networks as knowledge gatekeepers
Expert networks are specialized platforms that connect businesses with professionals who possess niche, high-value expertise – from retired industry leaders to active practitioners.
This is their core function, but these platforms have an enormous, yet unexplored, potential. They can structure expert engagements to extract that expertise before it disappears.
For businesses, these networks can act as knowledge cartographers. In a way, they are responsible for mapping domains where attrition poses the greatest risk.
They pinpoint retirees whose insights, such as decades of navigating regulatory mazes or troubleshooting obsolete machinery, are irreplaceable.
By curating vetted pools of experts, expert networks enable organizations to design systematic knowledge-transfer initiatives rather than relying on ad hoc mentorship.
Crucially, expert networks also serve as intermediaries between three parties:
- Businesses seeking future-proof operations
- Retiring experts who require frameworks to articulate their tacit knowledge
- AI trainers who need structured data to build context-aware models
Facilitating tacit and explicit knowledge capture
Expert networks can excel at capturing undocumented, experience-driven insights that resist digitization. Through structured interviews, shadowing, or scenario-based workshops, they are able to extract:
- Operational instincts, e.g., a retiring plant manager’s method of diagnosing equipment failures through sound or vibration.
- Crisis playbooks: Unwritten strategies to manage supply-chain collapses or PR disasters
- Relationship intelligence: How to navigate personalities in high-stakes negotiations.
Explicit Knowledge Transfer
Expert networks can also streamline the organization of codified data for AI ingestion:
- Legacy documentation: Scanning and tagging decades of manuals, SOPs, and reports to identify patterns
- Decision logs: Transforming historical choices (e.g., R&D investments, mergers) into labelled datasets.
- Compliance archives: Structuring regulatory submissions into case studies for AI analysis.
Frameworks for Knowledge Transfer Within Expert Networks
Human-AI collaboration models via expert networks
Expert networks enable businesses to deploy human-in-the-loop systems, where retiring experts validate and refine AI output during training. This iterative process ensures AI models absorb data and the reasoning behind human decisions.
These networks also foster hybrid knowledge bases – repositories that blend the insights of retiring experts with AI-generated patterns.
Think of it as a dynamic library whereby veterans contribute decades of decision-making precedents, while AI cross-references these with real-time data to detect predictive trends. Over time, guided by human expertise, the system learns to ask better questions.
Ontology-based knowledge capture
Ontologies – structured frameworks that define relationships between concepts in a domain – are vital to translate human expertise into AI-understandable formats. Expert networks help businesses to design these ontologies by:
- Identifying core concepts: Retiring experts can define key terms, processes, and decision-making criteria in their field.
- Mapping relationships: How do these concepts interact? For example, in manufacturing, a “machine fault” might be linked to vibration patterns, maintenance logs, and production delays.
- Validating hierarchies: Experts ensure the ontology reflects real-world priorities, not theoretical assumptions.
Knowledge distillation techniques
Expert networks support teacher-student AI architectures where retiring experts (teachers) train lightweight, deployable AI models (students). This distills complex expertise into efficient systems that retain critical decision-making logic.
The process involves:
- Expert-led training: Veterans demonstrate problem-solving in real-world scenarios (e.g., predicting market risks).
- Model simplification: AI trainers compress these lessons into smaller models without sacrificing accuracy.
- Continuous feedback: Experts audit student models’ outputs, correcting misinterpretations.
The bottom line
The race against the knowledge cliff demands more than urgency, it requires architecture. By leveraging expert networks as intermediaries, businesses can transform retiring expertise into AI-ready frameworks, bridging the gap between tacit wisdom and algorithmic precision.
The methodologies outlined here - human-AI collaboration models, ontology-based systems, and knowledge distillation - offer blueprints to preserve institutional memory while enhancing AI’s contextual intelligence. Yet this is only the foundation.
In the next article of the “knowledge transfer” series, we will expand on these frameworks, examining real-world implementations with case studies from industries already successfully scaling AI-trained expertise, metrics for ROI in knowledge transfer initiatives, and ethical considerations in automating human judgment.
The goal behind this knowledge transfer is not merely to archive expertise, but to evolve it to ensure that the synaptic sparks of one generation ignite the engines of the next.