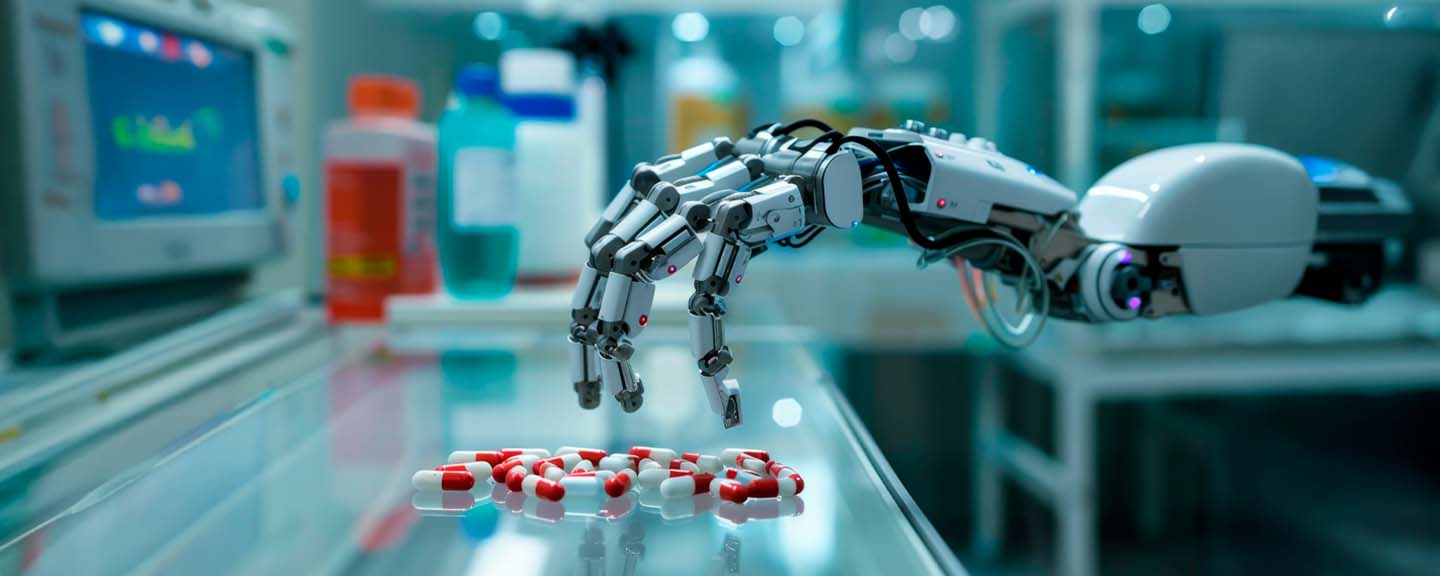
It is almost certain that drug discovery will accelerate due to the integration of artificial intelligence (AI).
In a nutshell, implementing AI algorithms ultimately helps the healthcare industry to save time and money and achieve much better results. In practice, AI is already significantly reducing the time required for certain chemical operations from months to hours.
This is why the use of AI in drug discovery can speed up the development of extremely important drugs such as those used for the treatment of cancer.
Furthermore, research released in 2024 found that the pharmaceuticals discovered by AI in phase 1 clinical trials have a higher success rate (80%–90%) than conventionally discovered drugs (40%–65%).
How exactly can AI assist in drug discovery?
1. Spotting disease-causing targets
AI algorithms can be used to examine complicated biological information and identify disease-causing targets such as proteins or genes.
With the help of machine learning algorithms, it is possible to streamline target validation to forecast how these targets and possible medication candidates would interact.
For example, AlphaFold – the AI program developed by DeepMind – has succeeded in reducing the protein structure prediction interval from months to hours, for which the tool’s developers, John Jumper and Demis Hassabis, were awarded the 2024 Nobel Prize in Chemistry.
Check out this inside look at the team from DeepMind that developed AlphaFold:
2. Predictive toxicology
By evaluating preclinical data, AI models can forecast the safety profile of medication candidates, reducing the possibility of unfavorable outcomes during human clinical trials.
Machine learning algorithms can detect toxicological patterns such as hepatotoxicity or cardiotoxicity, which enables the early exclusion of medication candidates that do not meet the safety criteria.
AI can improve clinical trials by helping to design better plans, predict outcomes, and group patients so that only those most likely to benefit from a treatment are included.
3. Using drugs for new indications
Repurposing current medications for new uses is another beneficial application of AI. It can be used to reveal the surprising benefits of some medications for unrelated diseases by evaluating enormous clinical and genomic datasets.
4. Enhanced pre-clinical trials
AI accelerates early-stage drug research by evaluating data from lab testing, such as experiments in test tubes or animals, to anticipate how well a possible treatment will work and how safe it is.
5. New drug design
AI can be used to alter drug design by creating unique molecular architectures that are optimized for both safety and effectiveness. How does this work?
It means AI can study enormous databases of known chemicals and how they interact with diseases to help to find new treatments for illnesses that currently have no cure.
6. Predictive modeling and simulation
AI can imitate the way intricate biological systems behave. With the help of this in silico technique, experts can forecast the effects of drugs at multiple stages, including absorption, distribution, and excretion.
AI in drug discovery: real-world successes
AI is revolutionizing medication research, speeding up development and lowering costs. Here are two real-world examples:
1. Drug against idiopathic pulmonary fibrosis
A Hong Kong-based company, Insilico Medicine, has been working on an experimental medication where generative AI is used to identify both the therapeutic compound and the biological target.
In March 2025, this clinical-stage GenAI-driven biotech company announced that the United States Adopted Names (USAN) Council had given its revolutionary drug candidate for idiopathic pulmonary fibrosis (IPF) ISM001-055, the official name Rentosertib.
2. Cancer-fighting drug
In April 2025, it was reported that scientists had used AI and advanced X-ray technology to find a promising new drug to fight several types of cancer.
AI aided in the rapid analysis of thousands of compounds to identify one that inhibits the enzyme AKR1C3, which is implicated in several types of cancer, including breast and prostate.
Ultimately, the study could result in improved cancer treatment plans with fewer adverse effects.
Challenges in AI-powered drug discovery research
The use of AI in the creation of medicines poses certain difficulties despite the technology having massive potential:
- Poor data quality – To function effectively, AI needs high-quality, comprehensive, and diverse datasets, but the data can often be incomplete or inconsistent, which compromises accuracy.
- Many AI systems work like a "black box" – While researchers can observe what enters and exits the AI algorithm, they are largely unaware of how AI makes a decision. This makes it difficult to trust the findings and determine who is at fault if something goes wrong, particularly in critical circumstances.
- Unclear rules and guidelines – Regulatory bodies are still getting used to the implementation of AI in drug development, and there may remain issues related to the setting of standards and best practices for AI validation and the moral use of patient data.
Final word:
There is no doubt that AI has great potential for drug discovery, being able to analyze huge datasets and provide rapid results, some of which may be very accurate (for now, we highlight the word “may”).
And this is where challenges also arise – sometimes the results could be incomplete and inconsistent, and scientists may not know how AI arrived at its decision.
However, with the evolution of technology, AI’s potential in drug development will continue to grow, and it is important to accept this fact, particularly in terms of strong collaboration among industry leaders, innovators, and regulators that can ensure ethical and effective solutions.